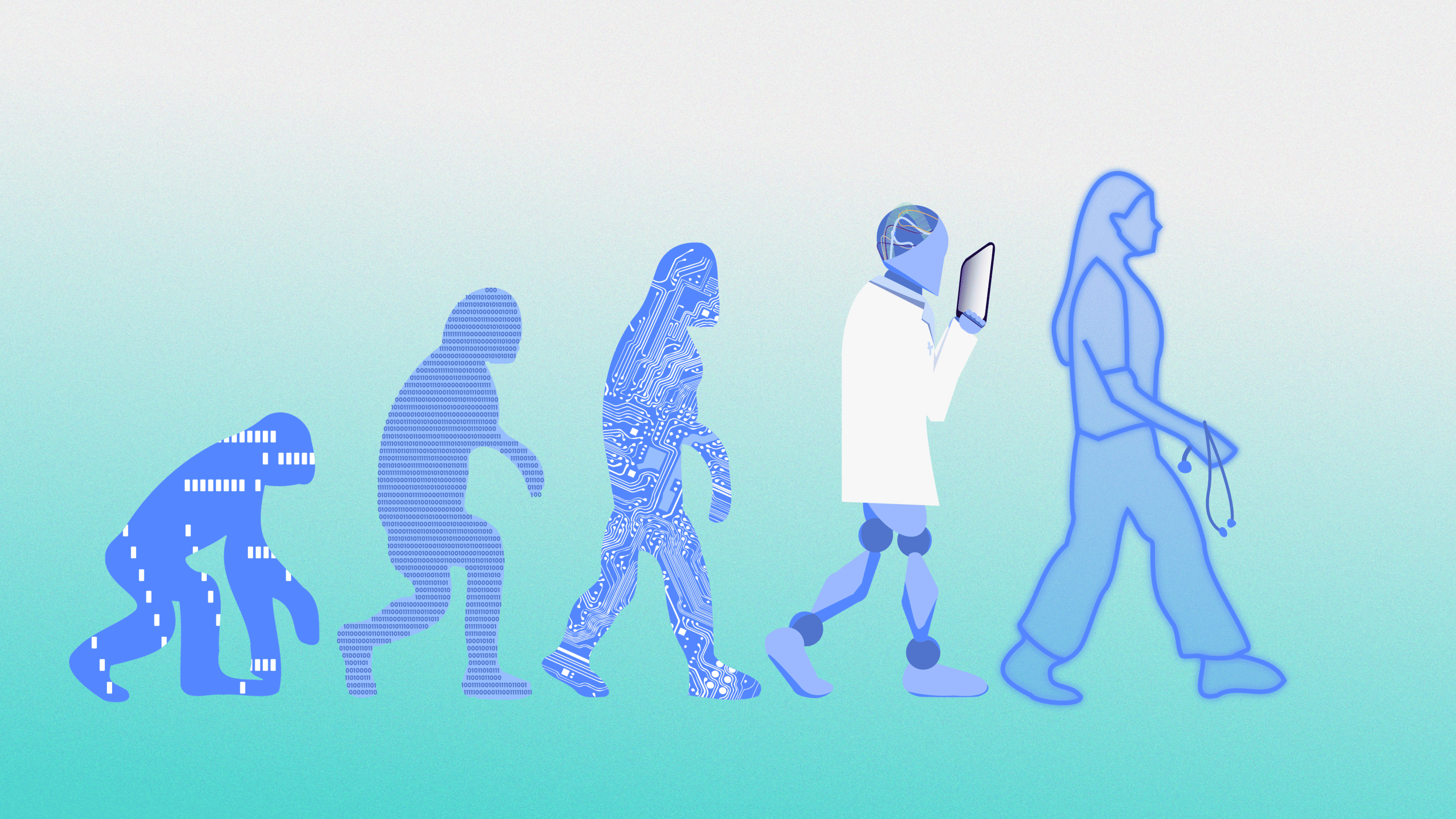
From Code to Cognition: Navigating AI’s Evolutionary Journey
Welcome to the first entry in Verto’s three-part blog series on AI in healthcare with Verto’s Director of AI & Technology Transformation, Martin Persaud. Martin kicks off the series with a brief chronology of AI evolution and catches us up to speed on some of the nomenclature and technologies under the AI umbrella.
Unless you have been living completely off the grid, you have probably seen more hype about artificial intelligence (AI) than just about anything else in the tech world this past year. While AI has been used for quite some time in everyday applications, (e.g., giving you personalized Spotify recommendations) it’s the new wave of generative AI, that has been causing the biggest splash.
However, the concept of AI isn’t as modern as one might think. In fact, the inception of AI as an independent concept occurred sometime in the 1950s, and we’ve been riding waves of hype ever since.
I’m not here to add to the hype train, so instead, I want to provide some context around how much (or how little) AI concepts have evolved through the years and why that is. I’d also like to set the stage for a more useful discussion, such as whether or not AI is ready to be used in critical industries such as healthcare, where each decision can literally become a matter of life and death.
Let’s start with a brief history of AI before we dig in further.
AI through the ages
The above timeline is not exhaustive but rather meant to represent a subset of key people and events that drove AI forward to its current state. The most important takeaway here is the general dates around the early establishment of AI and its strong resurgence in the past decade.
The AI Universe
Now we’re all on the same page on the rough history of AI. A couple of questions remain, though: How broad is the extent of the term “AI”? What does it mean to deploy AI technology?
We’ve created the infographic below to organize the terms and the types of algorithms that came with them.
As before, this infographic isn’t meant to be exhaustive, nor should it be treated as the source of truth for all things AI. However, it should help illustrate the many interrelated concepts that constitute AI. A few things from this image should jump out at you:
- AI is a broad term encompassing other technologies beyond a machine’s ability to learn. Rule-based systems still fall under this umbrella term.
- Traditional organization of machine learning concepts falls into the buckets of supervised, unsupervised, and reinforcement learning – We’ll touch on this later.
- Generative Adversarial Networks (‘GANs’) and Large Language Models (‘LLMs’) are just two of many deep learning methods and are major reasons for a lot of the hype around generative AI. Technologies like ChatGPT fall into this bucket and have brought AI to the mainstream in a way that has not happened in the past. AI now seems more accessible and applicable in everyday life.
When we look at the history of AI alongside its underlying algorithms, we start to see a shift from AI as a broad concept to a more narrow focus on deep learning.
A key nuance that everyone should consider is that while we try to place machine learning methods and algorithms in neat boxes (supervised, unsupervised, reinforcement learning), the reality is it is not so straightforward. It may be worth thinking about AI based on the type of task instead of the method. One simple approach could be whether a task requires one or a combination of the tasks below:
- Generative: The capability to create new content based on learned patterns.
- Predictive: The capability to make predictions about the future based on past events.
- Inferential: The capability to evaluate and analyze new information.
As AI is increasingly applied in healthcare, we generally make choices based on the definition of the task and whether layering these tasks can solve critical data and integration problems. We rarely discuss these choices in the context of supervised, unsupervised, and reinforcement learning, and perhaps we should.
Why this GEN-eration of AI is Different
It should be apparent by now that while core concepts of AI have been around for decades, we’ve seen exponential growth in both performance and complexity.Why is that?
Computational Power
One simple reason is the growth of brute-force computational power. Computational resources limited most early experimentation attempts, so they didn’t see the light of day until recently. For example, Moore’s Law observes that the number of transistors (electronic components directly impacting computing power) doubles every two years! The growth in transistors not only unlocks general computing power, it also contributes to the rise of parallel processing, where many specific tasks are simultaneously computed.
Data and Communication
Another key factor for the recent surge of AI is the growth in data collection, storage, and exchange. We are seeing greater than linear expansion of data creation and capture, allowing for an abundance of training data that did not exist the same way just 20 years ago. In most cases, simply providing more data for a machine to learn from will improve any model’s performance.
This graph, taken from Statista, depicts the total volume of data/information created, captured, copied, and consumed worldwide from 2010 to 2020, (including forecasts from 2021 to 2025).
What Does This All Mean?
The emergence of ChatGPT and other similar technologies seems like the beginnings of a silver bullet that trends toward general artificial intelligence. While that may excite (or scare) some of us, it may not be wise to make a bet that it will solve all of our problems today. In particular, when applied to industries like healthcare, we’re at a stage where we need to think a lot harder about the implications of the newfound generative AI wave. Here are some things we can be certain about:
- Size and Complexity: Algorithms, such as LLMs and the like, are computationally expensive to fine-tune and maintain. Move over Rolex and Balenciaga, being able to to deploy an LLM is the new mark of luxury. (GPU usage alone can cost upwards of $18,000 per month!).
- Trust and Confidence: When considering decisions about someone’s health, clinicians, patients, and other decision-makers need to be able to trust the information that generative models provide. A significant concern for many of these models are “hallucinations,” when LLMs detect nonexistent patterns and create inaccurate outputs.
- Interpretability: Users still want to understand an outcome – the answer itself is never enough. This is a particular concern in a healthcare setting.
- Continual Learning & Catastrophic Forgetting: As we apply AI in more complex settings, no two situations will be the same. Fine-tuning task-specific models to new environments will have to be closely monitored. The ability to continually learn while not forgetting (i.e., reducing performance on previous similar tasks) is a critical concern in enterprise use cases where a model needs to be applied across many client environments.
- Data Hygiene: While data is more widely collected / available, it is fraught with landmines around standardization and bias. This is a whole topic in itself that should be considered before a model is deployed.
This isn’t to say that new AI developments can’t make an impact now; quite the contrary. At Verto, we’re excited about the opportunities these developments present in solving problems in healthcare!
Looking Forward
Thanks for joining us for the first instalment of this series where we show you Verto’s take on AI! Looking forward, we’ll dive deeper into the context of AI in healthcare, covering some crucial topics like:
- Productionizing AI and the emerging field of MLOps
- The importance of data
- Key areas in healthcare where AI technology can make an impact today
Did this blog pique your interest? Check out the following links to learn more about the ever-evolving world of AI.
- For those that are just eager to learn more: https://www.vanderschaar-lab.com/
- For those that are interested in the challenges of healthcare (e.g., patient privacy, bias, the healthcare employment crisis, etc.): https://ml4h.cc/2023/
- For those that are interested in early applications of AI in healthcare (e.g., genomics expression, robotic surgery, etc.): https://drerictopol.com/portfolio/deep-medicine/